80% of projects fail because of data. Poor quality data. Why do we need AI & Data Governance?
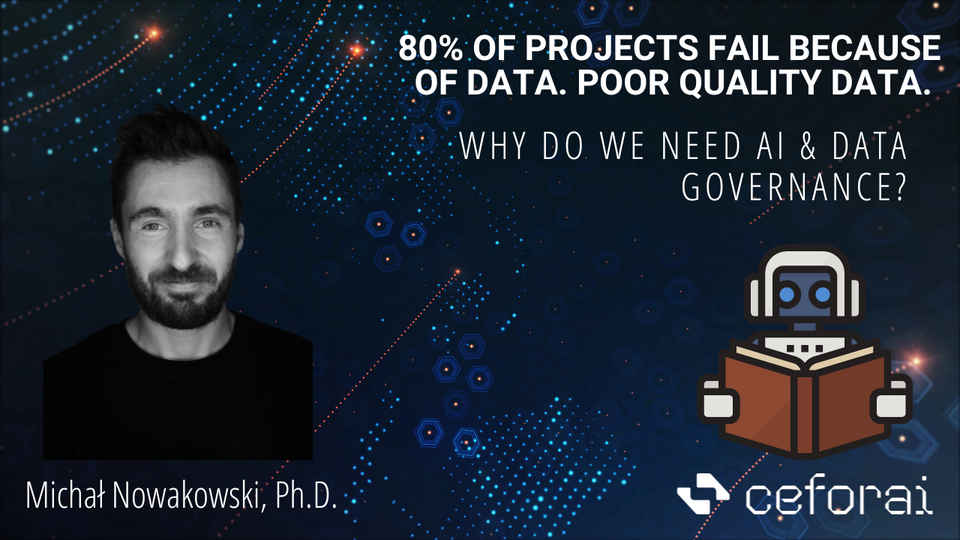
Data - the new oil of the digital world! The amount of data every day is growing almost exponentially! Data is changing the world! There is no other option than to start using data, which can come from various sources, to create better products and services. There's nothing left to do but roll up your sleeves, set a budget, and choose a project team, and in a few months, the first results will appear - either savings or real profits. Then all that's left to do is celebrate and get on with the next project. Well, the reality is not so rosy. According to Ataccama[1], as many as 88% of data integration projects fail (variously defined) or exceed (significantly) budgets. The reason was inadequate quality data, which further translated into the fact that as many as 33% of organizations abandoned or delayed the implementation of new IT systems for the same reason.
Unfortunately, deciding to use data, however crucial in the context of the "new approach" to digitization, is only the first step to implementing a data-driven project. According to McKinsey, by 2025 [2], about 463 exabytes will be "produced" every day (that's a lot), but the question is whether they will all be of the quality we would like. Data in itself is not necessarily valuable - as we look at it from above and building data-based products should be based on specific principles, which include feasibility (feasibility), value (practical), and usability (usable). Therefore, an excellent data-driven product will involve effective management of THIS specific project and result from good data management and analytics, or so-called #artificialintelligence.
Good quality data can be defined in many ways. One guideline can be found, for example, in Article 10(3) of the draft AI Act, which states that training, validation, and testing data sets must be adequate, representative, error-free and complete. They must have appropriate statistical properties, including, where applicable, for the individuals or groups against whom the high-risk AI system is to be used. Google experts point to such elements as (relative) errorless, timeliness, or accuracy. In practice, each organization should assess what will indicate that the data is of EXCELLENT quality to achieve the intended effect. The effect can also be measured in many ways. Without a doubt, however, ensuring that the data is "good" should be one of the goals of any organization that wants to be truly data-driven.
Why does it matter so much? You can find examples of poor-quality data use without looking far. Google, Apple, Youtube, and even Twitter are examples from the shore where it has been shown that improper selection and oversight of data sets used for training (and production) can result in severe consequences. And the discrimination I cited pretty often because of algorithmic bias is the only example of this. Suppose we are creating, for example, some predictive or decision-making model. In that case, if we do not provide adequate data, the result of the action can have disastrous consequences for us and others. Managing this area is therefore very important if we want to ensure that our product or service is also qualitatively good. And safe because we can't forget about data protection or privacy related to cyber security issues. Although in a very different sense than most data scientists understand, quality also ensures that data complies with legal and regulatory requirements, and GDPR is absolute.
This brings us to the question I posed in the title of today's column. Why does an organization need AI & Data Governance? To begin with, a definition is nothing more than a totality of organizational, technical, and human solutions to ensure that we have good quality products and services (including internal ones) in the organization. The Google experts I have already mentioned indicate that such a framework consists of the following:
• the area of so-called data discovery and evaluation of our data sets,
• classification of data and its organization,
• cataloging data and managing data about data or metadata,
• data quality management,
• controlling access to data,
• data auditing, and
• data protection.
Seemingly only seven points, but putting them into practice can require a lot of work, both at the solution design stage and on an ongoing basis. Unfortunately, building a competitive advantage with good quality data requires constant sacrifice, which ultimately translates into greater competitiveness, customer trust, and loyalty or reputation. Of course, implementing such a solution can also be part of meeting legal and regulatory requirements that are or will be imposed on more and more entities. Failure to meet them will likely result in liability in many fields. All the more so since new changes in #ai liability regulations or requirements for data intermediaries are already on the horizon.
We can, of course, assume that today there is no point in implementing new solutions or updating existing ones when there are no regulations linked to sanctions for not implementing them. In the long run, however, this is a somewhat risky approach for at least two reasons:
• Implementing AI & Data Governance is not an "invention" of the AI Act and related acts or regulatory requirements but is a necessary part of building a data-driven organization,
• Not having the right solutions is not only a compliance risk but, perhaps more importantly, a burn-through of money and resources and, just as often, a poor quality product and service.
So if we care about building a long-term strategy for a data-driven organization, then (at the very least) planning for change will take time too. This requires building a (new) culture and awareness and the right competencies. Suppose the organization needs to pay more attention to the value and risks of data processing. In that case, even if we provide a solid organizational and technical framework, it will fill the competence mentioned above the gap. Education is critical, but I have already written about that here. It is then essential to properly plan for these changes, which will require that we sit down and analyze the status quo in a broader group - not just those responsible (exclusively) for data.
Interdisciplinarity is our ally here, although reconciling seemingly divergent interests can be challenging for many leaders. Objectivity is also helpful in this regard, i.e., an external auditor who will know where the so-called bottlenecks may be. This is very important in the context of the new challenges that will await the organization.
Let’s not kid ourselves. It’s also a budget that will cover us in most of the areas that the entire AI & Data Governance covers, including strategy implementation. And let’s not fool ourselves again – how much we don’t plan will always be too little. However, resources must be provided if we are serious about such changes. We are looking from the perspective of future legal changes; if we invest today in adjusting to what may be in the future, it will lower the cost of adjustments for us when it becomes such a requirement. I know how difficult it is to convince the so-called C-level to make such an outlay. Still, if we point out that a „side effect” of transforming the organization into a data-driven organization is reducing compliance costs, it is only to our advantage.
So if we can make such a decision and move to implement it, we are getting closer to sizable savings and, in the long run, higher profits. In the U.S. alone, it is estimated that the savings of U.S. banks in operations related to the implementation of AI projected for 2023 will be about $7.3 billion. However, it seems this amount will be much higher. However, the work continues as there is an implementation phase ahead, which takes work and time. Especially if the organization has a "history" behind it; this, however, is the subject of a separate column. If you have questions about implementation planning or workshops, contact us.
Sources: https://www.ataccama.com/blog/the-cost-of-poor-data-quality
https://www.mckinsey.com/capabilities/mckinsey-digital/our-insights/data-ethics-what-it-means-and-what-it-takes
https://www.oreilly.com/library/view/data-mesh/9781492092384/
https://www.googleadservices.com/pagead/aclk?sa=L&ai=DChcSEwiVv_nXzLD7AhURBOYKHXJgCEUYABAPGgJscg&ohost=www.google.com&cid=CAESa-D2J5OMiIrK1z49TdKTpAyAulunDbg9fyrG1Ae8ohtNKRZfxUwM_tQwYJWbIIQkntpO7BjYdurxelMWVcdIElKmtvm9ulnT0A4igktm9T9DSRmfm5FrpPhsnD_Lo5RtRus_fno4-5MpaB0b&sig=AOD64_2uc3lvw-2t9NTradxNR6OBBfxA-g&ctype=5&q=&ved=2ahUKEwi89_DXzLD7AhXkiIsKHVTbBdwQwg8oAXoECAYQCw&adurl=